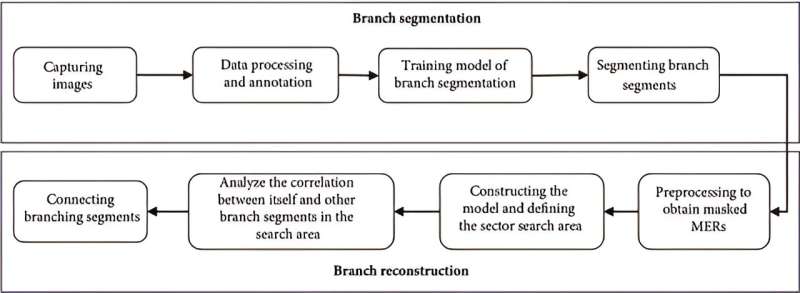
In traditional fruit production, formidable challenges arise from labor costs and shortages, prompting extensive research into agricultural automation and the use of intelligent robots for tasks like fruit picking and branch pruning.
Despite advancements in the detection and reconstruction of plant branches using both traditional vision techniques and 3D modeling, issues like occlusion, intricate natural environments, and the need for high-quality data persist. Recent studies leveraging deep learning have shown promise, with techniques like CNNs and Mask R-CNN improving the adaptability to complex backgrounds and the accuracy of branch reconstruction.
Nonetheless, further research is required to overcome environmental dependencies, reduce costs, and amplify the flexibility and accuracy of these technologies in actual orchard operations.
This study introduces a mask region-based convolutional neural network (Mask R-CNN) with deformable convolution to accurately segment branches in complex orchard backgrounds. The method was specifically enhanced to handle the intricate growth patterns and overlapping branches typical of vine-like fruit trees, such as passion fruit.
An innovative branch reconstruction algorithm with bidirectional sector search was employed to adaptively reconstruct the segmented branches, allowing for minor parameter adjustments and accommodating the irregular shapes and orientations of passion fruit tree branches. The results demonstrated the method’s efficacy, with the improved Mask R-CNN model achieving average precision, recall, and F1 scores of 64.30%, 76.51%, and 69.88%, respectively, for passion fruit branch detection.
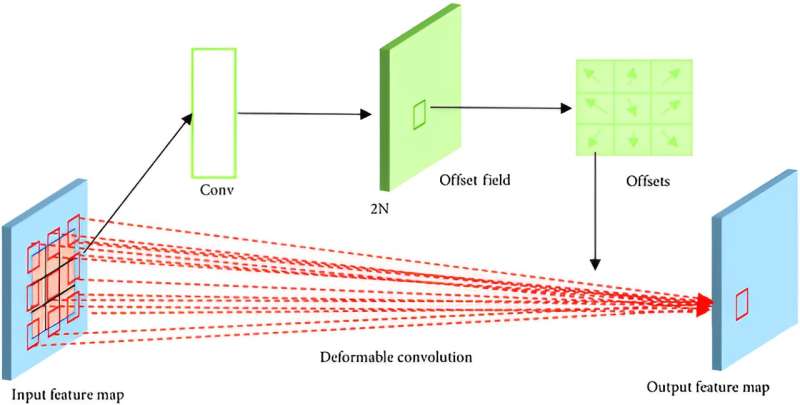
Notably, it outperformed the original Mask R-CNN and other comparative models, especially in complex lighting conditions. The branch reconstruction algorithm further illustrated the method’s robustness, with an 88.83% accuracy and 83.44% mean intersection-over-union (mIoU).
These figures underscore the model’s ability to precisely detect and reconstruct branches despite the challenging natural orchard environment. However, the study also acknowledges certain limitations and areas for improvement. While the method shows promise, issues like missed detections and false segmentations, especially for smaller or similarly colored branches, indicate the need for further refinement.
The model’s performance on various types of fruit trees also remains to be tested, indicating a potential direction for future research.
In conclusion, by integrating advanced deep learning techniques and an innovative reconstruction algorithm, the study offers a promising solution to the complexities of branch detection and reconstruction in natural orchard environments. This method not only advances the field of agricultural automation but also sets the stage for further improvements and adaptations to a wider range of agricultural applications.
The paper is published in the journal Plant Phenomics.
More information:
Jiangchuan Bao et al, Detection and Reconstruction of Passion Fruit Branches via CNN and Bidirectional Sector Search, Plant Phenomics (2023). DOI: 10.34133/plantphenomics.0088
Citation:
Advanced CNN techniques for accurate detection and reconstruction of passion fruit branches (2023, December 29)
retrieved 30 December 2023
from https://phys.org/news/2023-12-advanced-cnn-techniques-accurate-reconstruction.html
This document is subject to copyright. Apart from any fair dealing for the purpose of private study or research, no
part may be reproduced without the written permission. The content is provided for information purposes only.